Unleashing the Power of Data Annotation Tools and Platforms
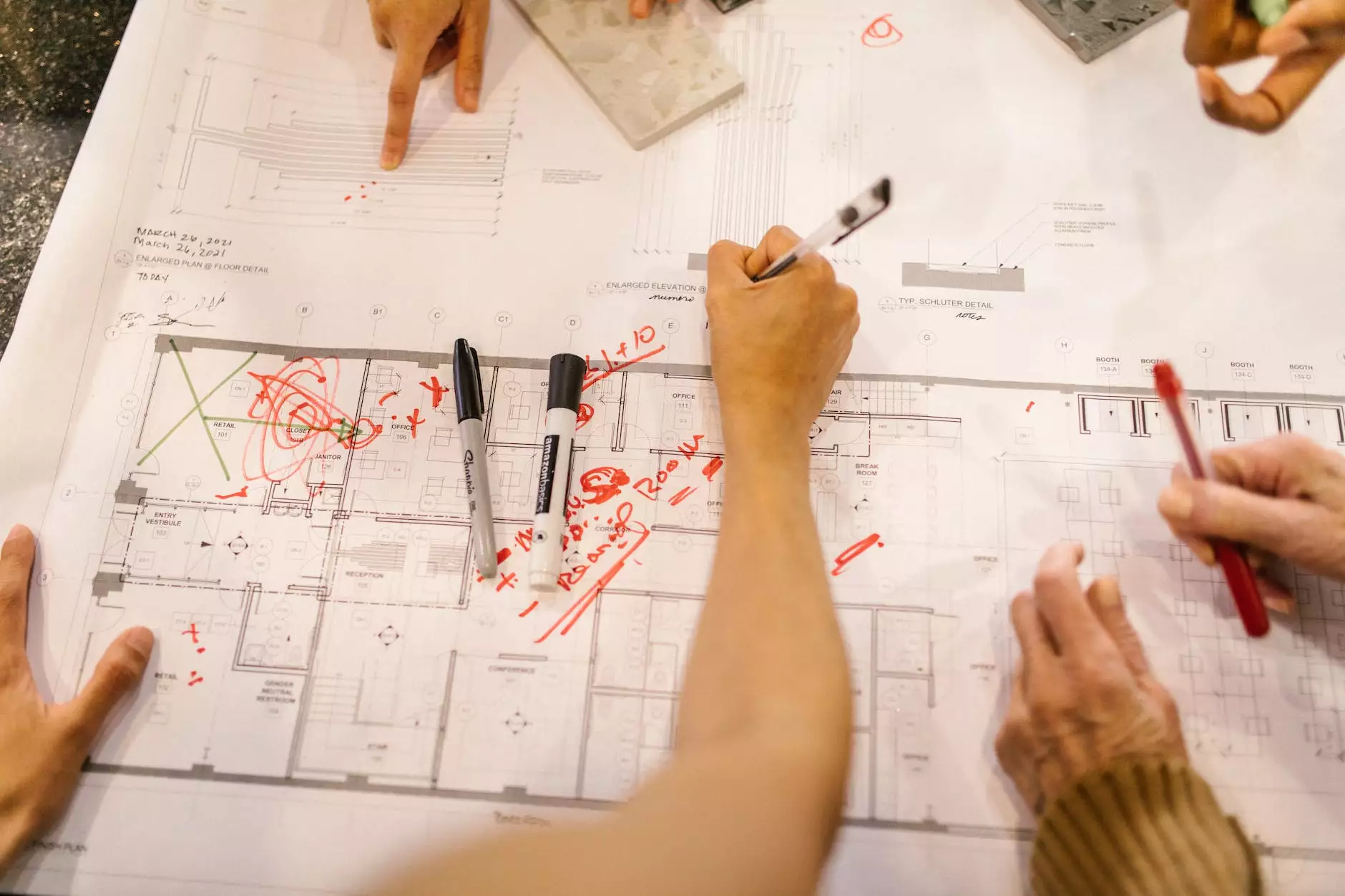
In today's digital age, where data-driven decisions shape the future of industries, the importance of reliable data annotation cannot be overstated. Every successful AI model starts with high-quality training data, and that is where data annotation tools and platforms, like those offered by https://keylabs.ai/, come into play.
Understanding Data Annotation
Data annotation is the process of labeling data to provide context for machine learning algorithms. This involves tagging multimedia content such as images, audio, and text to help AI systems identify relevant features. As organizations seek to refine their algorithms, the need for precise and efficient data annotation grows.
The Significance of Data Annotation
Quality data annotation is critical for several reasons:
- Improved Machine Learning Models: Accurate annotations ensure that machine learning models can learn and generalize better.
- Increased Efficiency: Automated processes can significantly reduce the time and effort involved in labeling data.
- High-Quality Results: The performance of AI systems, such as computer vision and natural language processing, heavily relies on the quality of annotated data.
Exploring Data Annotation Tools
Data annotation tools come in various shapes and sizes, catering to different needs and types of data. Here’s a look into the key features that make annotation tools effective:
1. User-Friendly Interfaces
Tools with intuitive user interfaces allow teams to jump right into the annotation process without extensive training. Features like drag-and-drop functionality, keyboard shortcuts, and on-screen instructions facilitate efficient workflows.
2. Versatile Annotation Types
Different projects require different types of annotations. A comprehensive tool should support:
- Image Annotation: Bounding boxes, polygons, and keypoints are crucial for object detection tasks.
- Text Annotation: Named entity recognition and sentiment analysis annotations are essential for NLP applications.
- Audio Annotation: Transcriptions and sound event labeling help in speech and audio interpretation.
3. Collaboration and Workflow Management
A robust data annotation platform should promote team collaboration. Features like task assignments, progress tracking, and real-time collaboration ensure that teams can work efficiently and keep projects on schedule.
4. Quality Assurance Mechanisms
To maintain high standards, data annotation tools must incorporate quality assurance practices. This could include:
- Automated validation checks.
- Peer review systems to ensure the accuracy of annotations.
- Metrics to analyze annotation quality and efficiency.
Benefits of a Professional Data Annotation Platform
Choosing a professional platform such as https://keylabs.ai/ can radically transform how businesses handle data annotation. Here are some clear benefits:
1. Scalability
As your project grows, the volume of data also increases. A professional platform can easily scale to accommodate more data without sacrificing quality.
2. Data Privacy and Security
With data breaches becoming increasingly common, using a reputable platform ensures that data is handled securely and complies with regulations such as GDPR.
3. Expert Support and Guidance
Platforms like KeyLabs AI provide expert support to assist businesses in improving their data strategies. This support can include customized training and resources tailored to the specific needs of the organization.
How to Choose the Right Data Annotation Tool
Faced with numerous data annotation tools available today, selecting the right one may seem daunting. Here are some essential factors to consider:
1. Project Requirements
Evaluate the specific needs of your project. Consider the volume of data, the types of data, and the complexity of the annotations needed. This understanding will help you select a tool tailored to your requirements.
2. Trial and Demonstration
Take advantage of trials or demonstrations. Most reputable platforms allow potential users to experience the tool firsthand, which can reveal if it aligns with your expectations.
3. Cost-Effectiveness
Ensure that the pricing structure fits your budget. Compare different platforms and analyze their offerings to find a solution that provides the best value for your investment.
4. Customer Reviews and References
Investigate customer reviews, testimonials, and case studies. Real-world experience from existing users can provide invaluable insights into the platform’s performance and reliability.
Implementing Data Annotation in Your Business Workflow
Integrating data annotation tools into your business processes can streamline operations significantly. Here's how:
1. Establish Clear Goals
Define what you want to achieve with your data annotation. Having clear objectives will guide your strategy and keep your team focused.
2. Process Definition
Outline the steps for your data annotation process, from data collection to the final review. Define roles for team members and ensure everyone understands their responsibilities.
3. Regular Training and Updates
As technology evolves, so should your team's skills. Regular training sessions on new tools and techniques will keep your team up to date and ensure consistent quality in annotations.
4. Iterate and Improve
Collect feedback on the annotation process and be willing to make adjustments. Continuous improvement is key to achieving better outcomes and more refined data sets over time.
Case Studies: Success with Data Annotation
Let’s explore how businesses have leveraged platforms like https://keylabs.ai/ to achieve tangible results:
1. E-commerce Platform
An e-commerce company utilized a data annotation platform to label product images. This effort improved their visual search function, resulting in a 30% increase in user engagement and a significant boost in sales.
2. Healthcare AI Application
A healthcare startup used advanced data annotation tools to label medical images for training their diagnostic AI. The result was a more accurate model, enhancing patient care and establishing trust with stakeholders.
3. Autonomous Vehicle Development
A self-driving car company collected vast amounts of data from vehicle cameras. By using a robust annotation platform, they successfully tagged objects in diverse environments, leading to safer navigation algorithms.
Conclusion: The Future of Data Annotation
The role of data annotation in the advancement of AI and machine learning cannot be underestimated. As the digital landscape continues to evolve, businesses must adapt and harness the benefits of top-notch annotation tools and platforms. This is more than just a trend but rather a necessity for any company aiming to keep pace with innovation.
By leveraging the capabilities offered by platforms like https://keylabs.ai/, organizations can ensure that their machine learning models are trained with the best data possible, leading to enhanced outcomes and increased competitiveness in their respective markets.
In summary, data annotation tools and platforms are integral to building robust AI systems that can transform industries. Investing in quality annotation solutions is not just about enhancing your business’s analytical capabilities, but it’s also about preparing for the future of intelligent technology.